Behind the scenes at the Conflict Analytics Lab, all our tools are powered by machine learning algorithms. These algorithms are fueled by well-organized data. Collecting, analyzing and organizing the data that we use to fuel those machine learning algorithms is a huge chunk of the work that we do. This year we are turning things a bit upside-down and using our machine learning skills to make our data analysis better.
Before getting into the improvements we are trying to make, it would be worth a quick peek behind the curtain to see how new tools are made.
After deciding on an access-to-justice tool to build, which is a whole other blog post, we:
1) Find all the court decisions that answered questions the tool is looking to answer.
2) Analyze the cases to extract relevant details, for example, duration of employment or amount of notice given.
3) Convert the details into a machine-readable form such as numbers or checkmarks in a spreadsheet.
4) Process that data with the machine learning algorithms to generate insights
This is our data pipeline. It involves dozens of law students analyzing hundreds of cases. If we could improve our pipeline to make lives easier for our analysts, it would have an enormous impact on how quickly we can develop these tools.
This year we took on two improvements to that pipeline.
The first improvement is a collaboration with LIDI (https://lidi.ca/) which gave us access to the text of around 500,000 court and tribunal decisions. Before we had this repository, we would have to either download the cases by hand or do the analysis one at a time online. We still managed to analyze hundreds of cases this way, but it limited what we could do with our machine learning chops.
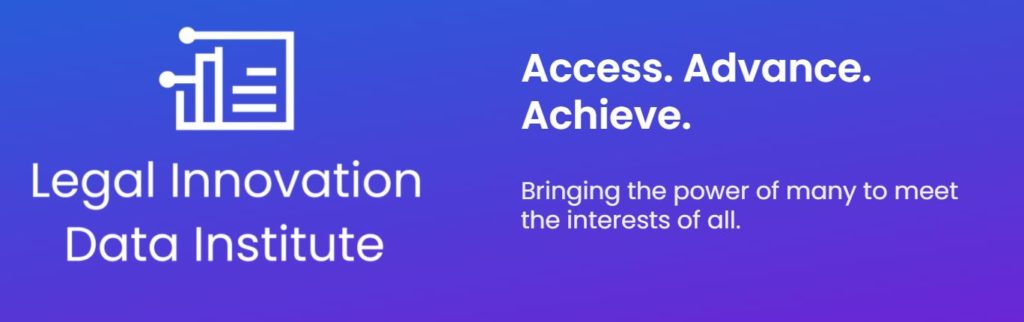
As a result of having easy access to those decisions, we can try to lighten the load on our analysts with some computer-aided legal analysis. While we are still in the early stages of developing these improvements, they are already showing promise. We are leveraging natural language processing techniques to highlight likely-relevant sections of the cases. Even simple named-entity recognition has shown great promise and we are expecting to find that more sophisticated analysis will yield even more optimization. This is potentially very exciting. With hundreds of cases to analyze for any given project, any optimization will be a big boon and a massive time savings.
If you are a researcher interested in getting involved or have any questions, feel free to reach out at contact@conflict-analytics.org
(Photo by Matthew Henry from Burst (https://burst.shopify.com)